
Welcome to the Computational Turbulence Laboratory (CTLab) at KU!
Our Vision
We study turbulent flows over complex surfaces in both low and high Mach number regimes. Our expertise encompasses Computational Fluid Dynamics, High-Performance Computing, and Artificial Intelligence. By developing high-order numerical schemes and employing state-of-the-art theoretical and data-driven methods, we aim to understand and predict the aero-thermo-acoustic characteristics of turbulent flows over complex surfaces, such as wall roughness and porous media. Our work advances supersonic aerothermodynamics, clean energy harvesting, and naval engineering.
Supersonic Aerothermodynamics
We study high-Mach number boundary layers over rough walls to examine the impact of shock-boundary layer-roughness interactions on the turbulence field and heat transfer. The end goal is to shed light on roughness-induced early transition to turbulence and surface ablation phenomena, and devise turbulence models to predict roughness effects in extreme compressible regimes. Additionally, we explore the potential use of anisotropic porous media for turbulence control in high-Mach number flows.
Below is a video of periodic turbulent channel flow over a rough surface at Mach 1.5 — the first, and probably the only, visualization of such flow simulations.
Clean Energy Harvesting
Our work contributes to the optimization of wind turbine blades by understanding surface corrosion-induced roughness and mitigating its effects through passive turbulence control using porous materials. We explored porous media applications in flow control, noise reduction, delaying transition to turbulence and stabilizing vortex shedding around bluff-bodies.
We analyzed turbulent boundary layers featuring a separation bubble (shown below). Our results indicate that the characteristics of the separation bubble —such as its size, turbulence intensity and acoustic noise level— significantly depend on, and can be influenced by, the underlying porous media geometry.
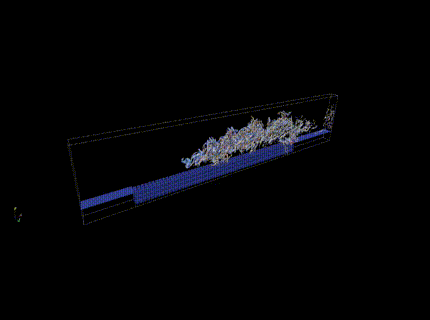
To understand the effects of roughness on wind turbine blade aerodynamics, we studied the turbulence structures of rough-wall channel flow over a realistic turbine blade surface and compared the results with those of synthetic rough walls (sandgrain and cube) and a smooth surface. Linear stochastic estimation suggests that near the wall, the quasi-streamwise vortices observed in smooth-wall flow are present in the large-scale recessed regions of turbine blade roughness, whereas in sandgrain and cube roughness, they are replaced by 'head-up, head-down' horseshoe structures. Our results highlight the sensitivity of turbulent coherent motions to roughness texture, particularly within the roughness sublayer.
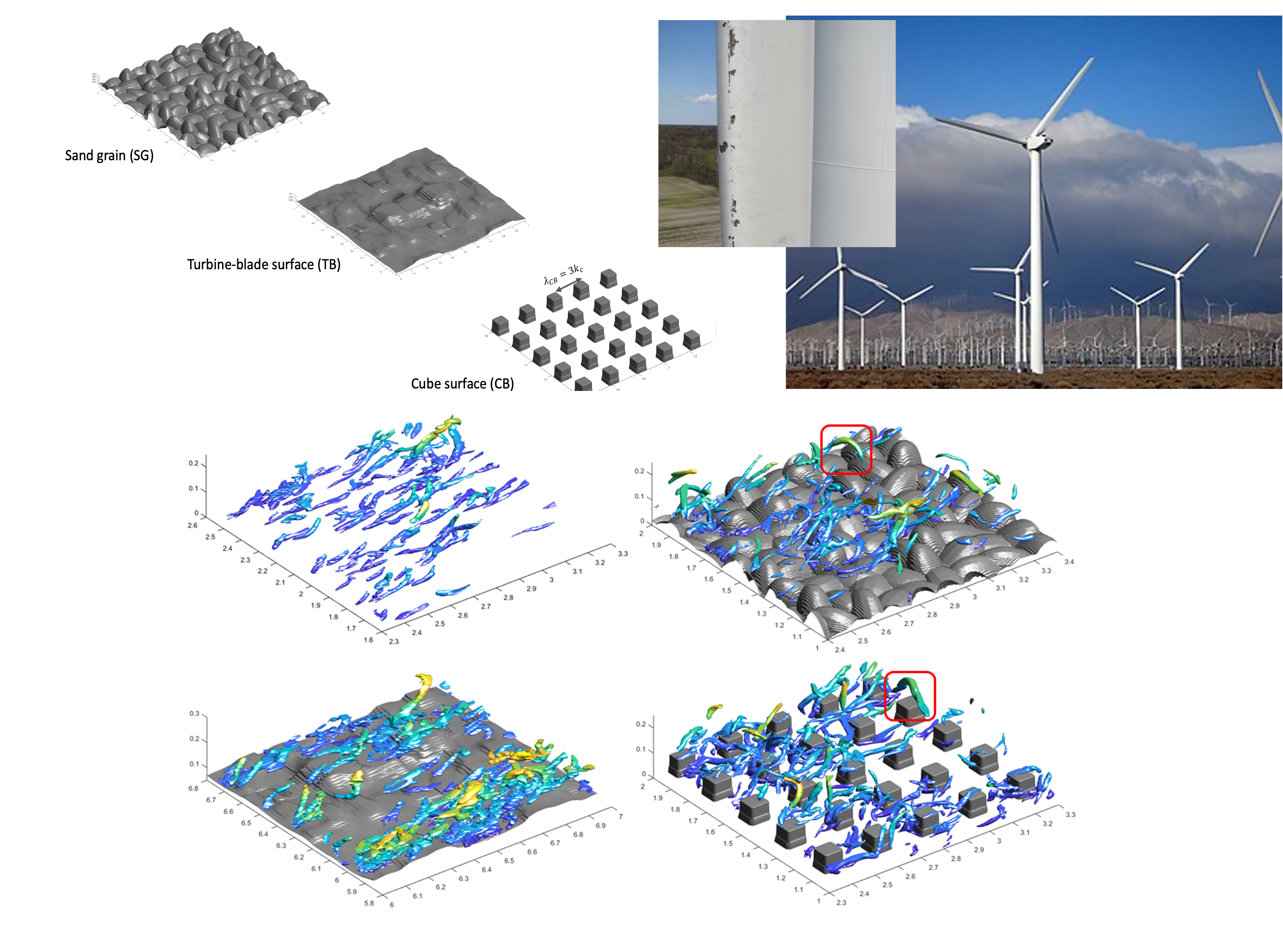
AI-Driven Naval Applications
The bodies and propeller blades of naval vessels erode in the saline oceans environment. This erosion causes surface roughness, which increases the hydrodynamic drag while lessening the vessels longevity. Additionally, they produce hydrodynamic noise, which compromises the stealth capabilities of naval vessels and submarines. We utilize machine learning and AI to accurately predict flow behavior over rough-wall turbulent boundary layers across diverse flow conditions. These conditions may encompass non-equilibrium flows and flows over curved surfaces, enhancing the current state-of-the-art in rough-wall aerohydrodynamics.
Our pioneering work, as demonstrated by our publication in Aghaei-Jouybari, et. al. (2021), J. Fluid Mech. 912, A8, represents the very first attempt to predict the roughness equivalent sandgrain height (ks) using AI. ks is a key hydrodynamic parameter that characterizes both the hydrodynamic drag and the underwater radiated noise level. Generating a comprehensive DNS database, consisting of 45 surfaces (24 of them shown below), we designed a neural network that predicts ks with less than 10% error — a significant improvement compared to the existing correlations.
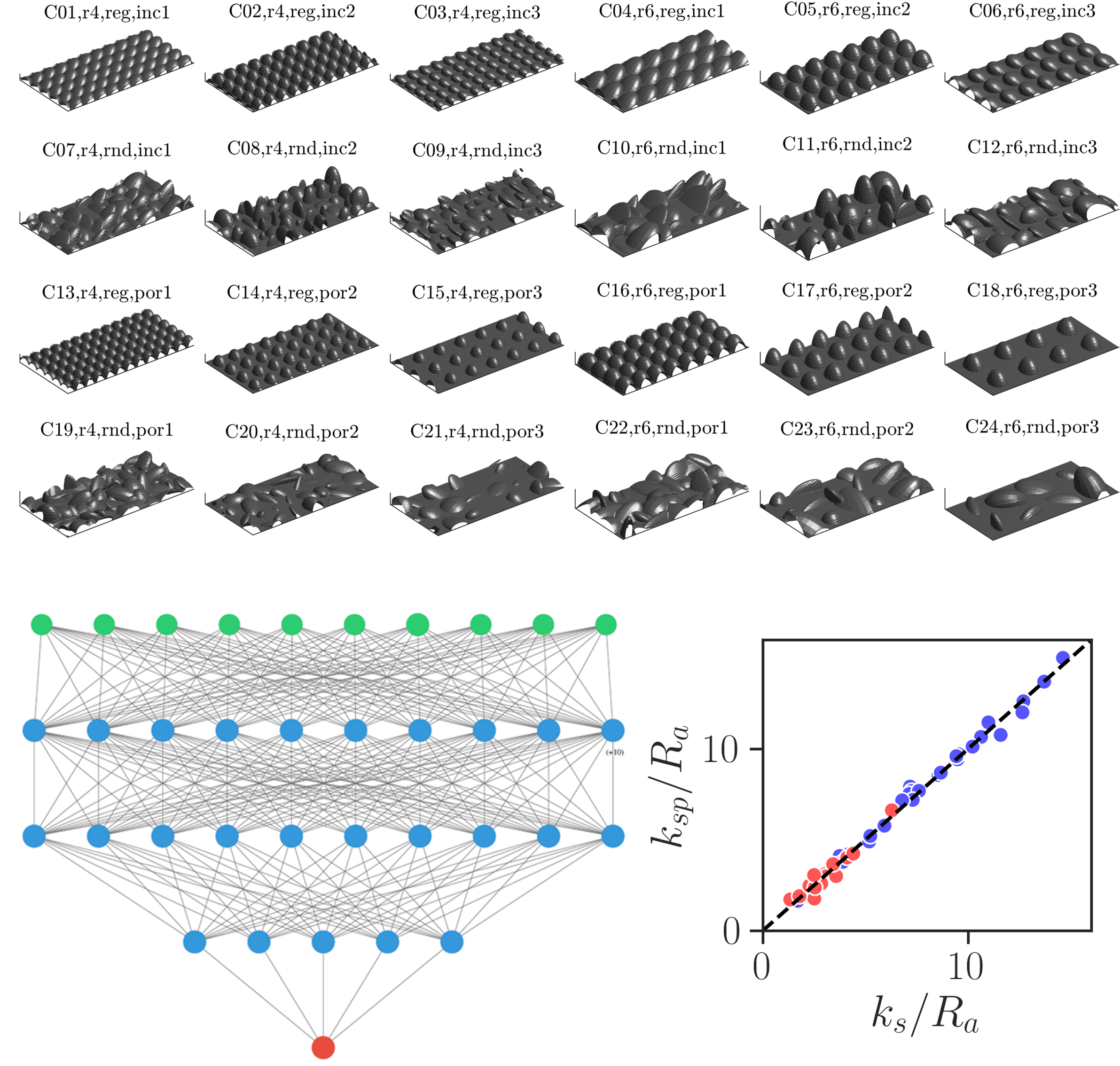